Open Access
ARTICLE
Image Denoising with Adaptive Weighted Graph Filtering
1 School of Information Science and Engineering, Southeast University, Nanjing, 210096, China.
2 Department of Psychiatry and Translational Imaging, Columbia University & NYSPI, New York, 10032, USA.
3 College of Internet of Things Engineering, Hohai University, Changzhou, 213022, China.
4 Changzhou Key Laboratory of Sensor Networks and Environmental Sensing, Changzhou, 213022, China.
* Corresponding Author: Li Zhao. Email: .
Computers, Materials & Continua 2020, 64(2), 1219-1232. https://doi.org/10.32604/cmc.2020.010638
Received 15 March 2020; Accepted 20 April 2020; Issue published 10 June 2020
Abstract
Graph filtering, which is founded on the theory of graph signal processing, is proved as a useful tool for image denoising. Most graph filtering methods focus on learning an ideal lowpass filter to remove noise, where clean images are restored from noisy ones by retaining the image components in low graph frequency bands. However, this lowpass filter has limited ability to separate the low-frequency noise from clean images such that it makes the denoising procedure less effective. To address this issue, we propose an adaptive weighted graph filtering (AWGF) method to replace the design of traditional ideal lowpass filter. In detail, we reassess the existing low-rank denoising method with adaptive regularizer learning (ARLLR) from the view of graph filtering. A shrinkage approach subsequently is presented on the graph frequency domain, where the components of noisy image are adaptively decreased in each band by calculating their component significances. As a result, it makes the proposed graph filtering more explainable and suitable for denoising. Meanwhile, we demonstrate a graph filter under the constraint of subspace representation is employed in the ARLLR method. Therefore, ARLLR can be treated as a special form of graph filtering. It not only enriches the theory of graph filtering, but also builds a bridge from the low-rank methods to the graph filtering methods. In the experiments, we perform the AWGF method with a graph filter generated by the classical graph Laplacian matrix. The results show our method can achieve a comparable denoising performance with several state-of-the-art denoising methods.Keywords
Cite This Article
Citations
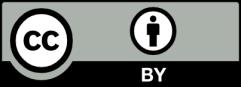
This work is licensed under a Creative Commons Attribution 4.0 International License , which permits unrestricted use, distribution, and reproduction in any medium, provided the original work is properly cited.