Open Access
ARTICLE
Three-Phase Unbalance Prediction of Electric Power Based on Hierarchical Temporal Memory
1 School of Automation and Electrical Engineering, University of Science and Technology Beijing, Beijing,
100083, China.
2 School of Computer and Communication Engineering, University of Science and Technology Beijing, Beijing, 100083, China.
3 Beijing Key Laboratory of Knowledge Engineering for Materials Science, Beijing, 100083, China.
4 Amphenol Assemble Tech, Houston, TX 77070, USA.
* Corresponding Author: Aziguli Wulamu. Email: .
Computers, Materials & Continua 2020, 64(2), 987-1004. https://doi.org/10.32604/cmc.2020.09812
Received 20 January 2020; Accepted 14 April 2020; Issue published 10 June 2020
Abstract
The difference in electricity and power usage time leads to an unbalanced current among the three phases in the power grid. The three-phase unbalanced is closely related to power planning and load distribution. When the unbalance occurs, the safe operation of the electrical equipment will be seriously jeopardized. This paper proposes a Hierarchical Temporal Memory (HTM)-based three-phase unbalance prediction model consisted by the encoder for binary coding, the spatial pooler for frequency pattern learning, the temporal pooler for pattern sequence learning, and the sparse distributed representations classifier for unbalance prediction. Following the feasibility of spatialtemporal streaming data analysis, we adopted this brain-liked neural network to a real-time prediction for power load. We applied the model in five cities (Tangshan, Langfang, Qinhuangdao, Chengde, Zhangjiakou) of north China. We experimented with the proposed model and Long Short-term Memory (LSTM) model and analyzed the predict results and real currents. The results show that the predictions conform to the reality; compared to LSTM, the HTM-based prediction model shows enhanced accuracy and stability. The prediction model could serve for the overload warning and the load planning to provide high-quality power grid operation.Keywords
Cite This Article
Citations
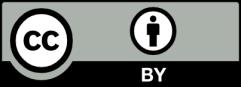
This work is licensed under a Creative Commons Attribution 4.0 International License , which permits unrestricted use, distribution, and reproduction in any medium, provided the original work is properly cited.