Open Access
ARTICLE
GACNet: A Generative Adversarial Capsule Network for Regional Epitaxial Traffic Flow Prediction
1 National Pilot School of Software, Yunnan University, Kunming, 650091, China.
2 School of Engineering and Applied Science, The George Washington University, Washington DC, 20052, USA.
* Corresponding Author: Yan Kang. Email: .
Computers, Materials & Continua 2020, 64(2), 925-940. https://doi.org/10.32604/cmc.2020.09903
Received 25 January 2020; Accepted 28 February 2020; Issue published 10 June 2020
Abstract
With continuous urbanization, cities are undergoing a sharp expansion within the regional space. Due to the high cost, the prediction of regional traffic flow is more difficult to extend to entire urban areas. To address this challenging problem, we present a new deep learning architecture for regional epitaxial traffic flow prediction called GACNet, which predicts traffic flow of surrounding areas based on inflow and outflow information in central area. The method is data-driven, and the spatial relationship of traffic flow is characterized by dynamically transforming traffic information into images through a two-dimensional matrix. We introduce adversarial training to improve performance of prediction and enhance the robustness. The generator mainly consists of two parts: abstract traffic feature extraction in the central region and traffic prediction in the extended region. In particular, the feature extraction part captures nonlinear spatial dependence using gated convolution, and replaces the maximum pooling operation with dynamic routing, finally aggregates multidimensional information in capsule form. The effectiveness of the method is evaluated using traffic flow datasets for two real traffic networks: Beijing and New York. Experiments on highly challenging datasets show that our method performs well for this task.Keywords
Cite This Article
Citations
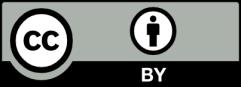
This work is licensed under a Creative Commons Attribution 4.0 International License , which permits unrestricted use, distribution, and reproduction in any medium, provided the original work is properly cited.