Open Access
ARTICLE
Performance Anomaly Detection in Web Services: An RNN- Based Approach Using Dynamic Quality of Service Features
1 School of Information Technology, Monash University, Subang Jaya, 47500, Malaysia.
2 Graduate School of Business IT, Kookmin University, Seoul, Korea.
3 School of Information Technology, Monash University, Subang Jaya, 47500, Malaysia.
4 Department of Mathematical and Computer Sciences, Indiana University of Pennsylvania, Indiana, USA.
* Corresponding Author: Seung Ryul Jeong. Email: .
Computers, Materials & Continua 2020, 64(2), 729-752. https://doi.org/10.32604/cmc.2020.010394
Received 02 March 2020; Accepted 09 April 2020; Issue published 10 June 2020
Abstract
Performance anomaly detection is the process of identifying occurrences that do not conform to expected behavior or correlate with other incidents or events in time series data. Anomaly detection has been applied to areas such as fraud detection, intrusion detection systems, and network systems. In this paper, we propose an anomaly detection framework that uses dynamic features of quality of service that are collected in a simulated setup. Three variants of recurrent neural networks-SimpleRNN, long short term memory, and gated recurrent unit are evaluated. The results reveal that the proposed method effectively detects anomalies in web services with high accuracy. The performance of the proposed anomaly detection framework is superior to that of existing approaches using maximum accuracy and detection rate metrics.Keywords
Cite This Article
Citations
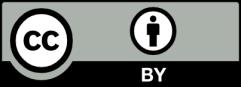
This work is licensed under a Creative Commons Attribution 4.0 International License , which permits unrestricted use, distribution, and reproduction in any medium, provided the original work is properly cited.