Open Access
ARTICLE
Corpus Augmentation for Improving Neural Machine Translation
1 University of Science and Technology Liaoning, Anshan, 114031, China.
2 College of Science & Health, Technological University Dublin, Dublin, D08 X622, Ireland.
* Corresponding Author: Chengying Chi. Email: ;
Computers, Materials & Continua 2020, 64(1), 637-650. https://doi.org/10.32604/cmc.2020.010265
Received 21 February 2020; Accepted 11 April 2020; Issue published 20 May 2020
Abstract
The translation quality of neural machine translation (NMT) systems depends largely on the quality of large-scale bilingual parallel corpora available. Research shows that under the condition of limited resources, the performance of NMT is greatly reduced, and a large amount of high-quality bilingual parallel data is needed to train a competitive translation model. However, not all languages have large-scale and high-quality bilingual corpus resources available. In these cases, improving the quality of the corpora has become the main focus to increase the accuracy of the NMT results. This paper proposes a new method to improve the quality of data by using data cleaning, data expansion, and other measures to expand the data at the word and sentence-level, thus improving the richness of the bilingual data. The long short-term memory (LSTM) language model is also used to ensure the smoothness of sentence construction in the process of sentence construction. At the same time, it uses a variety of processing methods to improve the quality of the bilingual data. Experiments using three standard test sets are conducted to validate the proposed method; the most advanced fairseq-transformer NMT system is used in the training. The results show that the proposed method has worked well on improving the translation results. Compared with the state-of-the-art methods, the BLEU value of our method is increased by 2.34 compared with that of the baseline.Keywords
Cite This Article
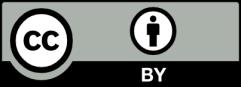
This work is licensed under a Creative Commons Attribution 4.0 International License , which permits unrestricted use, distribution, and reproduction in any medium, provided the original work is properly cited.