Open Access
ARTICLE
Quantum Generative Adversarial Network: A Survey
1 School of Cybersecurity, Chengdu University of Information Technology, Chengdu, 610225, China.
2 International Business Machines Corporation, New York, 14201, USA.
* Corresponding Author: Shibin Zhang. Email: .
Computers, Materials & Continua 2020, 64(1), 401-438. https://doi.org/10.32604/cmc.2020.010551
Received 09 March 2020; Accepted 31 March 2020; Issue published 20 May 2020
Abstract
Generative adversarial network (GAN) is one of the most promising methods for unsupervised learning in recent years. GAN works via adversarial training concept and has shown excellent performance in the fields image synthesis, image super-resolution, video generation, image translation, etc. Compared with classical algorithms, quantum algorithms have their unique advantages in dealing with complex tasks, quantum machine learning (QML) is one of the most promising quantum algorithms with the rapid development of quantum technology. Specifically, Quantum generative adversarial network (QGAN) has shown the potential exponential quantum speedups in terms of performance. Meanwhile, QGAN also exhibits some problems, such as barren plateaus, unstable gradient, model collapse, absent complete scientific evaluation system, etc. How to improve the theory of QGAN and apply it that have attracted some researcher. In this paper, we comprehensively and deeply review recently proposed GAN and QAGN models and their applications, and we discuss the existing problems and future research trends of QGAN.Keywords
Cite This Article
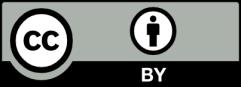
This work is licensed under a Creative Commons Attribution 4.0 International License , which permits unrestricted use, distribution, and reproduction in any medium, provided the original work is properly cited.