Open Access
ARTICLE
Influence Diffusion Model in Multiplex Networks
1 School of Computer Science and Technology, Nanjing University of Aeronautics and Astronautics, Nanjing, 210000, China.
2 School of Computer and Information, Shanghai Second Polytechnic University, Shanghai, China.
3 Department of Infection, Immunity and Inflammation, University of Leicester, Leicester, LE1 7RH, UK.
* Corresponding Author: Senbo Chen. Email: .
Computers, Materials & Continua 2020, 64(1), 345-358. https://doi.org/10.32604/cmc.2020.09807
Received 19 January 2020; Accepted 04 March 2020; Issue published 20 May 2020
Abstract
The problem of influence maximizing in social networks refers to obtaining a set of nodes of a specified size under a specific propagation model so that the aggregation of the node-set in the network has the greatest influence. Up to now, most of the research has tended to focus on monolayer network rather than on multiplex networks. But in the real world, most individuals usually exist in multiplex networks. Multiplex networks are substantially different as compared with those of a monolayer network. In this paper, we integrate the multi-relationship of agents in multiplex networks by considering the existing and relevant correlations in each layer of relationships and study the problem of unbalanced distribution between various relationships. Meanwhile, we measure the distribution across the network by the similarity of the links in the different relationship layers and establish a unified propagation model. After that, place on the established multiplex network propagation model, we propose a basic greedy algorithm on it. To reduce complexity, we combine some of the characteristics of triggering model into our algorithm. Then we propose a novel MNStaticGreedy algorithm which is based on the efficiency and scalability of the StaticGreedy algorithm. Our experiments show that the novel model and algorithm are effective, efficient and adaptable.Keywords
Cite This Article
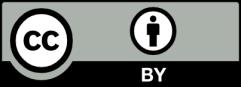
This work is licensed under a Creative Commons Attribution 4.0 International License , which permits unrestricted use, distribution, and reproduction in any medium, provided the original work is properly cited.