Open Access
ARTICLE
A New Sequential Image Prediction Method Based on LSTM and DCGAN
1 School of Computer & Software, Jiangsu Engineering Center of Network Monitoring, Nanjing University of Information Science & Technology, Nanjing, 210044, China.
2 State Key Laboratory for Novel Software Technology, Nanjing University, Nanjing, 210023, China.
3 Department of Economics, Finance, Insurance and Risk Management University of Central Arkansas, Conway, 72035, USA.
* Corresponding Author: Feihong Zhang. Email: .
Computers, Materials & Continua 2020, 64(1), 217-231. https://doi.org/10.32604/cmc.2020.06395
Received 18 February 2019; Accepted 08 April 2019; Issue published 20 May 2020
Abstract
Image recognition technology is an important field of artificial intelligence. Combined with the development of machine learning technology in recent years, it has great researches value and commercial value. As a matter of fact, a single recognition function can no longer meet people’s needs, and accurate image prediction is the trend that people pursue. This paper is based on Long Short-Term Memory (LSTM) and Deep Convolution Generative Adversarial Networks (DCGAN), studies and implements a prediction model by using radar image data. We adopt a stack cascading strategy in designing network connection which can control of parameter convergence better. This new method enables effective learning of image features and makes predictive models to have greater generalization capabilities. Experiments demonstrate that our network model is more robust and efficient in terms of timing prediction than 3DCNN and traditional ConvLSTM. The sequential image prediction model architecture proposed in this paper is theoretically applicable to all sequential images.Keywords
Cite This Article
Citations
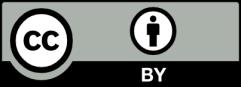
This work is licensed under a Creative Commons Attribution 4.0 International License , which permits unrestricted use, distribution, and reproduction in any medium, provided the original work is properly cited.