Open Access
ARTICLE
Analysis of Semi-Supervised Text Clustering Algorithm on Marine Data
1 College of Computer Science and Technology, Jilin University, Changchun, 130012, China.
2 A Key Laboratory of Symbolic Computation and Knowledge Engineering, Jilin University, Changchun, 130012, China.
3 Department of Engineering Mechanics, State Marine Technical University of St. Petersburg, St. Petersburg, 190008, Russia.
* Corresponding Author: Lili He. Email: .
Computers, Materials & Continua 2020, 64(1), 207-216. https://doi.org/10.32604/cmc.2020.09861
Received 22 January 2020; Accepted 12 February 2020; Issue published 20 May 2020
Abstract
Semi-supervised clustering improves learning performance as long as it uses a small number of labeled samples to assist un-tagged samples for learning. This paper implements and compares unsupervised and semi-supervised clustering analysis of BOAArgo ocean text data. Unsupervised K-Means and Affinity Propagation (AP) are two classical clustering algorithms. The Election-AP algorithm is proposed to handle the final cluster number in AP clustering as it has proved to be difficult to control in a suitable range. Semi-supervised samples thermocline data in the BOA-Argo dataset according to the thermocline standard definition, and use this data for semi-supervised cluster analysis. Several semi-supervised clustering algorithms were chosen for comparison of learning performance: Constrained-K-Means, Seeded-K-Means, SAP (Semi-supervised Affinity Propagation), LSAP (Loose Seed AP) and CSAP (Compact Seed AP). In order to adapt the single label, this paper improves the above algorithms to SCKM (improved Constrained-K-Means), SSKM (improved Seeded-K-Means), and SSAP (improved Semi-supervised Affinity Propagationg) to perform semi-supervised clustering analysis on the data. A DSAP (Double Seed AP) semi-supervised clustering algorithm based on compact seeds is proposed as the experimental data shows that DSAP has a better clustering effect. The unsupervised and semi-supervised clustering results are used to analyze the potential patterns of marine data.Keywords
Cite This Article
Citations
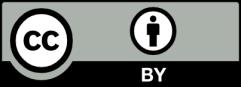
This work is licensed under a Creative Commons Attribution 4.0 International License , which permits unrestricted use, distribution, and reproduction in any medium, provided the original work is properly cited.