Open Access
ARTICLE
A Strategy of Signal Detection for Performance Improvement in Clipping Based OFDM System
1 Department of Information and Communication Engineering, Sejong University, Seoul, Korea.
2 Department of IT Contents, Hanshin University, Osan-si, Korea.
3 Department of System Semiconductor Engineering, SangMyung University, Cheonan-si, 31066, Korea.
* Corresponding Author: Hyoung-Kyu Song. Email: .
Computers, Materials & Continua 2020, 64(1), 181-191. https://doi.org/10.32604/cmc.2020.09998
Received 03 February 2020; Accepted 04 April 2020; Issue published 20 May 2020
Abstract
In this paper, the supervised Deep Neural Network (DNN) based signal detection is analyzed for combating with nonlinear distortions efficiently and improving error performances in clipping based Orthogonal Frequency Division Multiplexing (OFDM) ssystem. One of the main disadvantages for the OFDM is the high Peak to Average Power Ratio (PAPR). The clipping is a simple method for the PAPR reduction. However, an effect of the clipping is nonlinear distortion, and estimations for transmitting symbols are difficult despite a Maximum Likelihood (ML) detection at the receiver. The DNN based online signal detection uses the offline learning model where all weights and biases at fullyconnected layers are set to overcome nonlinear distortions by using training data sets. Thus, this paper introduces the required processes for the online signal detection and offline learning, and compares error performances with the ML detection in the clipping-based OFDM systems. In simulation results, the DNN based signal detection has better error performance than the conventional ML detection in multi-path fading wireless channel. The performance improvement is large as the complexity of system is increased such as huge Multiple Input Multiple Output (MIMO) system and high clipping rate.Keywords
Cite This Article
Citations
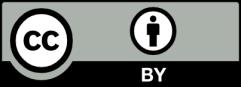
This work is licensed under a Creative Commons Attribution 4.0 International License , which permits unrestricted use, distribution, and reproduction in any medium, provided the original work is properly cited.