Open Access
ARTICLE
Resource Allocation and Power Control Policy for Device-toDevice Communication Using Multi-Agent Reinforcement Learning
1 Beijing Key Laboratory of Work Safety Intelligent Monitoring, Beijing University of Posts and
Telecommunications, Beijing, 100876, China.
2 Alibaba Cloud Computing, Hangzhou, 311121, China.
3 Department of Systems and Computer Engineering, Carleton University, Ottawa, K1S 5B6, Canada.
* Corresponding Author: Yifei Wei. Email: .
Computers, Materials & Continua 2020, 63(3), 1515-1532. https://doi.org/10.32604/cmc.2020.09130
Received 13 November 2019; Accepted 01 March 2020; Issue published 30 April 2020
Abstract
Device-to-Device (D2D) communication is a promising technology that can reduce the burden on cellular networks while increasing network capacity. In this paper, we focus on the channel resource allocation and power control to improve the system resource utilization and network throughput. Firstly, we treat each D2D pair as an independent agent. Each agent makes decisions based on the local channel states information observed by itself. The multi-agent Reinforcement Learning (RL) algorithm is proposed for our multi-user system. We assume that the D2D pair do not possess any information on the availability and quality of the resource block to be selected, so the problem is modeled as a stochastic non-cooperative game. Hence, each agent becomes a player and they make decisions together to achieve global optimization. Thereby, the multi-agent Q-learning algorithm based on game theory is established. Secondly, in order to accelerate the convergence rate of multi-agent Q-learning, we consider a power allocation strategy based on Fuzzy Cmeans (FCM) algorithm. The strategy firstly groups the D2D users by FCM, and treats each group as an agent, and then performs multi-agent Q-learning algorithm to determine the power for each group of D2D users. The simulation results show that the Q-learning algorithm based on multi-agent can improve the throughput of the system. In particular, FCM can greatly speed up the convergence of the multi-agent Q-learning algorithm while improving system throughput.Keywords
Cite This Article
Citations
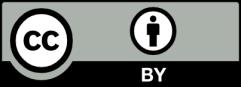
This work is licensed under a Creative Commons Attribution 4.0 International License , which permits unrestricted use, distribution, and reproduction in any medium, provided the original work is properly cited.