Open Access
ARTICLE
Joint Deep Matching Model of OCT Retinal Layer Segmentation
1 School of Information Science and Engineering at Shandong Normal University, Jinan, 250358, China.
2 Key Lab of Intelligent Computing and Information Security in Universities of Shandong, Shandong
Provincial Key Laboratory for Novel Distributed Computer Software Technology, Institute of Biomedical
Sciences, Shandong Normal University, Jinan, 250358, China.
3 INSA Lyon, University of Lyon, CNRS, Inserm, Villeurbanne, 69621 Cedex, France.
* Corresponding Authors: Yuanjie Zheng. Email: ;
Weikuan Jia. Email: .
Computers, Materials & Continua 2020, 63(3), 1485-1498. https://doi.org/10.32604/cmc.2020.09940
Received 30 January 2020; Accepted 01 March 2020; Issue published 30 April 2020
Abstract
Optical Coherence Tomography (OCT) is very important in medicine and provide useful diagnostic information. Measuring retinal layer thicknesses plays a vital role in pathophysiologic factors of many ocular conditions. Among the existing retinal layer segmentation approaches, learning or deep learning-based methods belong to the state-of-art. However, most of these techniques rely on manual-marked layers and the performances are limited due to the image quality. In order to overcome this limitation, we build a framework based on gray value curve matching, which uses depth learning to match the curve for semi-automatic segmentation of retinal layers from OCT. The depth convolution network learns the column correspondence in the OCT image unsupervised. The whole OCT image participates in the depth convolution neural network operation, compares the gray value of each column, and matches the gray value sequence of the transformation column and the next column. Using this algorithm, when a boundary point is manually specified, we can accurately segment the boundary between retinal layers. Our experimental results obtained from a 54-subjects database of both normal healthy eyes and affected eyes demonstrate the superior performances of our approach.Keywords
Cite This Article
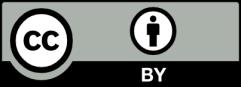
This work is licensed under a Creative Commons Attribution 4.0 International License , which permits unrestricted use, distribution, and reproduction in any medium, provided the original work is properly cited.