Open Access
ARTICLE
An Efficient Image Analysis Framework for the Classification of Glioma Brain Images Using CNN Approach
1 Faculty of Electrical Engineering, Botswana International University of Science and Technology, Palapye, Botswana.
2 Faculty of Computer Science Engineering, Vivekanandha College of Engineering for Women, Namakkal, 637205, India.
3 Department of Computer Networking, Lebanese French University, Erbil, 44001, Iraq.
4 Institute of Applied Sciences, University of Sciences, Techniques and Technologies of Bamako, Bamako, Mali.
* Corresponding Author: Ravi Samikannu. Email: .
Computers, Materials & Continua 2020, 63(3), 1133-1142. https://doi.org/10.32604/cmc.2020.08578
Received 10 September 2019; Accepted 12 January 2020; Issue published 30 April 2020
Abstract
The identification of brain tumors is multifarious work for the separation of the similar intensity pixels from their surrounding neighbours. The detection of tumors is performed with the help of automatic computing technique as presented in the proposed work. The non-active cells in brain region are known to be benign and they will never cause the death of the patient. These non-active cells follow a uniform pattern in brain and have lower density than the surrounding pixels. The Magnetic Resonance (MR) image contrast is improved by the cost map construction technique. The deep learning algorithm for differentiating the normal brain MRI images from glioma cases is implemented in the proposed method. This technique permits to extract the linear features from the brain MR image and glioma tumors are detected based on these extracted features. Using k-mean clustering algorithm the tumor regions in glioma are classified. The proposed algorithm provides high sensitivity, specificity and tumor segmentation accuracy.Keywords
Cite This Article
Citations
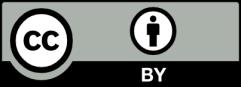
This work is licensed under a Creative Commons Attribution 4.0 International License , which permits unrestricted use, distribution, and reproduction in any medium, provided the original work is properly cited.