Open Access
ARTICLE
Improvement of Stochastic Competitive Learning for Social Network
1 School of Information Technology and Cyber Security, People’s Public Security University of China, Beijing, 100038, China.
* Corresponding Author: Yijun Gu. Email: ;
.
Computers, Materials & Continua 2020, 63(2), 755-768. https://doi.org/10.32604/cmc.2020.07984
Received 17 July 2019; Accepted 06 August 2019; Issue published 01 May 2020
Abstract
As an unsupervised learning method, stochastic competitive learning is commonly used for community detection in social network analysis. Compared with the traditional community detection algorithms, it has the advantage of realizing the timeseries community detection by simulating the community formation process. In order to improve the accuracy and solve the problem that several parameters in stochastic competitive learning need to be pre-set, the author improves the algorithms and realizes improved stochastic competitive learning by particle position initialization, parameter optimization and particle domination ability self-adaptive. The experiment result shows that each improved method improves the accuracy of the algorithm, and the F1 score of the improved algorithm is 9.07% higher than that of original algorithm.Keywords
Cite This Article
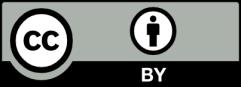
This work is licensed under a Creative Commons Attribution 4.0 International License , which permits unrestricted use, distribution, and reproduction in any medium, provided the original work is properly cited.