Open Access
ARTICLE
Cold Start Problem of Vehicle Model Recognition under Cross-Scenario Based on Transfer Learning
1 State Key Laboratory of Networking and Switching Technology, Beijing University of Posts and Telecommunications, Beijing, 100876, China.
2 National Engineering Laboratory for Public Safety Risk Perception and Control, China Academy of Electronics and Information Technology, Beijing, 100041, China.
3 University of Louisville, Louisville, KY, 40292, US.
* Corresponding Author: Hongbo Wang. Email: .
Computers, Materials & Continua 2020, 63(1), 337-351. https://doi.org/10.32604/cmc.2020.07290
Received 13 May 2019; Accepted 10 June 2019; Issue published 30 March 2020
Abstract
As a major function of smart transportation in smart cities, vehicle model recognition plays an important role in intelligent transportation. Due to the difference among different vehicle models recognition datasets, the accuracy of network model training in one scene will be greatly reduced in another one. However, if you don’t have a lot of vehicle model datasets for the current scene, you cannot properly train a model. To address this problem, we study the problem of cold start of vehicle model recognition under cross-scenario. Under the condition of small amount of datasets, combined with the method of transfer learning, load the DAN (Deep Adaptation Networks) and JAN (Joint Adaptation Networks) domain adaptation modules into the convolutional neural network AlexNet and ResNet, and get four models: AlexNet-JAN, AlexNet-DAN, ResNet-JAN, and ResNet-DAN which can achieve a higher accuracy at the beginning. Through experiments, transfer the vehicle model recognition from the network image dataset (source domain) to the surveillance-nature dataset (target domain), both Top-1 and Top-5 accuracy have been improved by at least 20%.Keywords
Cite This Article
Citations
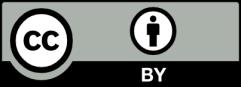
This work is licensed under a Creative Commons Attribution 4.0 International License , which permits unrestricted use, distribution, and reproduction in any medium, provided the original work is properly cited.