Open Access
ARTICLE
A Rub-Impact Recognition Method Based on Improved Convolutional Neural Network
1 College of Civil Aviation, Nanjing University of Aeronautics and Astronautics, Nanjing, 210016, China.
2 School of Information Engineering, Nanjing Audit University, Nanjing, 211815, China.
3 National Engineering Research Center of Turbo-generator Vibration, Southeast University, Nanjing, 210009, China.
* Corresponding Author: Weibo Yang. Email: .
Computers, Materials & Continua 2020, 63(1), 283-299. https://doi.org/10.32604/cmc.2020.07511
Received 29 May 2019; Accepted 01 July 2019; Issue published 30 March 2020
Abstract
Based on the theory of modal acoustic emission (AE), when the convolutional neural network (CNN) is used to identify rotor rub-impact faults, the training data has a small sample size, and the AE sound segment belongs to a single channel signal with less pixel-level information and strong local correlation. Due to the convolutional pooling operations of CNN, coarse-grained and edge information are lost, and the top-level information dimension in CNN network is low, which can easily lead to overfitting. To solve the above problems, we first propose the use of sound spectrograms and their differential features to construct multi-channel image input features suitable for CNN and fully exploit the intrinsic characteristics of the sound spectra. Then, the traditional CNN network structure is improved, and the outputs of all convolutional layers are connected as one layer constitutes a fused feature that contains information at each layer, and is input into the network’s fully connected layer for classification and identification. Experiments indicate that the improved CNN recognition algorithm has significantly improved recognition rate compared with CNN and dynamical neural network (DNN) algorithms.Keywords
Cite This Article
Citations
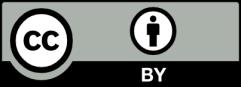