Open Access
ARTICLE
A Novel Bidirectional LSTM and Attention Mechanism Based Neural Network for Answer Selection in Community Question Answering
1 College of Information, Mechanical and Electrical Engineering, Shanghai Normal University, Shanghai, 200234, China.
2 The Computer Science and Computer Engineering Department, University of Arkansas, Fayetteville, AR, 72703, USA.
# The author contributes equally to this work and should be considered co-first author.
* Corresponding Author: Meizi Li. Email: .
Computers, Materials & Continua 2020, 62(3), 1273-1288. https://doi.org/10.32604/cmc.2020.07269
Abstract
Deep learning models have been shown to have great advantages in answer selection tasks. The existing models, which employ encoder-decoder recurrent neural network (RNN), have been demonstrated to be effective. However, the traditional RNN-based models still suffer from limitations such as 1) high-dimensional data representation in natural language processing and 2) biased attentive weights for subsequent words in traditional time series models. In this study, a new answer selection model is proposed based on the Bidirectional Long Short-Term Memory (Bi-LSTM) and attention mechanism. The proposed model is able to generate the more effective question-answer pair representation. Experiments on a question answering dataset that includes information from multiple fields show the great advantages of our proposed model. Specifically, we achieve a maximum improvement of 3.8% over the classical LSTM model in terms of mean average precision.Keywords
Cite This Article
Citations
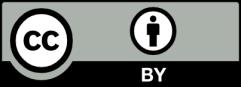
This work is licensed under a Creative Commons Attribution 4.0 International License , which permits unrestricted use, distribution, and reproduction in any medium, provided the original work is properly cited.