Open Access
ARTICLE
Empirical Comparisons of Deep Learning Networks on Liver Segmentation
1 School of Electronic and Information Engineering, Suzhou University of Science and Technology, Suzhou, China.
2 Department of Computer Science, University of Central Arkansas, Conway, Arkansas, USA.
3 Huan Provincial Key Laboratory of Intelligent Processing of Big Data on Transportation, School of Computer
and Communication Engineering, Changsha University of Science and Technology, Changsha, China.
* Corresponding Author: Victor S. Sheng. Email: .
Computers, Materials & Continua 2020, 62(3), 1233-1247. https://doi.org/10.32604/cmc.2020.07450
Abstract
Accurate segmentation of CT images of liver tumors is an important adjunct for the liver diagnosis and treatment of liver diseases. In recent years, due to the great improvement of hard device, many deep learning based methods have been proposed for automatic liver segmentation. Among them, there are the plain neural network headed by FCN and the residual neural network headed by Resnet, both of which have many variations. They have achieved certain achievements in medical image segmentation. In this paper, we firstly select five representative structures, i.e., FCN, U-Net, Segnet, Resnet and Densenet, to investigate their performance on liver segmentation. Since original Resnet and Densenet could not perform image segmentation directly, we make some adjustments for them to perform live segmentation. Our experimental results show that Densenet performs the best on liver segmentation, followed by Resnet. Both perform much better than Segnet, U-Net, and FCN. Among Segnet, U-Net, and FCN, U-Net performs the best, followed by Segnet. FCN performs the worst.Keywords
Cite This Article
Citations
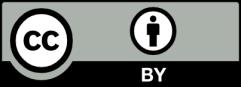
This work is licensed under a Creative Commons Attribution 4.0 International License , which permits unrestricted use, distribution, and reproduction in any medium, provided the original work is properly cited.