Open Access
ARTICLE
A Novel Combinational Convolutional Neural Network for Automatic Food-Ingredient Classification
1 College of Computer Science and Information Technology, Central South University of Forestry & Technology, Changsha, 410114, China.
2 School of Computing and Information Sciences, Florida International University, Miami, FL 33199, USA.
* Corresponding Author: Cong Li. Email: .
Computers, Materials & Continua 2020, 62(2), 731-746. https://doi.org/10.32604/cmc.2020.06508
Abstract
With the development of deep learning and Convolutional Neural Networks (CNNs), the accuracy of automatic food recognition based on visual data have significantly improved. Some research studies have shown that the deeper the model is, the higher the accuracy is. However, very deep neural networks would be affected by the overfitting problem and also consume huge computing resources. In this paper, a new classification scheme is proposed for automatic food-ingredient recognition based on deep learning. We construct an up-to-date combinational convolutional neural network (CBNet) with a subnet merging technique. Firstly, two different neural networks are utilized for learning interested features. Then, a well-designed feature fusion component aggregates the features from subnetworks, further extracting richer and more precise features for image classification. In order to learn more complementary features, the corresponding fusion strategies are also proposed, including auxiliary classifiers and hyperparameters setting. Finally, CBNet based on the well-known VGGNet, ResNet and DenseNet is evaluated on a dataset including 41 major categories of food ingredients and 100 images for each category. Theoretical analysis and experimental results demonstrate that CBNet achieves promising accuracy for multi-class classification and improves the performance of convolutional neural networks.Keywords
Cite This Article
Citations
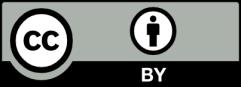