Open Access
ARTICLE
A General Framework of Reversible Data Hiding with Controlled Contrast Enhancement
1 School of Information Engineering, Guangdong University of Technology, Guangzhou, 510006, China.
2 Guangdong Key Laboratory of Intelligent Information Processing and Shenzhen Key Laboratory of Media
Security, Shenzhen University, 518060, China.
3 Department of Electrical and Computer Engineering, New Jersey Institute of Technology, Newark, NJ 07103 USA.
4 College of Computer Science and Electronic Engineering, Hunan University, Changsha, 410082, China.
5 School of Information Engineering, Xizang Minzu University, Xianyang, 712082, China
6 School of Electronic Information, Qingdao University, Qingdao, 266071, China.
* Corresponding Author: Shaowei Weng. Email: .
Computers, Materials & Continua 2020, 62(1), 157-177. https://doi.org/10.32604/cmc.2020.05681
Abstract
This paper proposes a two-step general framework for reversible data hiding (RDH) schemes with controllable contrast enhancement. The first step aims at preserving visual perception as much as possible on the basis of achieving high embedding capacity (EC), while the second step is used for increasing image contrast. In the second step, some peak-pairs are utilized so that the histogram of pixel values is modified to perform histogram equalization (HE), which would lead to the image contrast enhancement. However, for HE, the utilization of some peak-pairs easily leads to over-enhanced image contrast when a large number of bits are embedded. Therefore, in our proposed framework, contrast over-enhancement is avoided by controlling the degree of contrast enhancement. Since the second step can only provide a small amount of data due to controlled contrast enhancement, the first one helps to achieve a large amount of data without degrading visual quality. Any RDH method which can achieve high EC while preserve good visual quality, can be selected for the first step. In fact, Gao et al.’s method is a special case of our proposed framework. In addition, two simple and commonly-used RDH methods are also introduced to further demonstrate the generalization of our framework.Keywords
Cite This Article
Citations
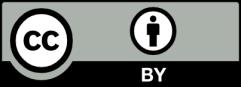