Open Access
ARTICLE
Hashtag Recommendation Using LSTM Networks with Self-Attention
1 School of Computer and Information Engineering, Henan University, Kaifeng, 475000, China.
2 The Corporate and Investment, Bank Technology, Canary Wharf, London, E145JP, UK.
* Corresponding Author: Jun Sun. Email: sunjunjerry@outlook.com.
Computers, Materials & Continua 2019, 61(3), 1261-1269. https://doi.org/10.32604/cmc.2019.06104
Abstract
On Twitter, people often use hashtags to mark the subject of a tweet. Tweets have specific themes or content that are easy for people to manage. With the increase in the number of tweets, how to automatically recommend hashtags for tweets has received wide attention. The previous hashtag recommendation methods were to convert the task into a multi-class classification problem. However, these methods can only recommend hashtags that appeared in historical information, and cannot recommend the new ones. In this work, we extend the self-attention mechanism to turn the hashtag recommendation task into a sequence labeling task. To train and evaluate the proposed method, we used the real tweet data which is collected from Twitter. Experimental results show that the proposed method can be significantly better than the most advanced method. Compared with the state-of-the-art methods, the accuracy of our method has been increased 4%.Keywords
Cite This Article
Citations
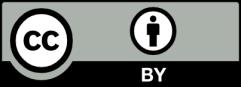
This work is licensed under a Creative Commons Attribution 4.0 International License , which permits unrestricted use, distribution, and reproduction in any medium, provided the original work is properly cited.