Open Access
ARTICLE
MMLUP: Multi-Source & Multi-Task Learning for User Profiles in Social Network
1 School of Computer Science and Technology, Harbin Institute of Technology, Weihai, 264209, China.
2 College of Information Engineering, Sanming University, 365004, Sanming, China.
3 Fujian Province University Key Lab for Industry Big Data Analysis and Application, Fujian, China.
4 College of Information Engineering, Qingdao Binhai University, Qingdao, China.
5 Jiangsu Province Wireless Sensing System Application Engneering Technology Research and Development Centre, China.
6 School of Engineering, Manukau Institute of Technology, Auckland, 2241, New Zealand.
* Corresponding Author: Chuiju You. Email: youchuiju@126.com.
Computers, Materials & Continua 2019, 61(3), 1105-1115. https://doi.org/10.32604/cmc.2019.06041
Abstract
With the rapid development of the mobile Internet, users generate massive data in different forms in social network every day, and different characteristics of users are reflected by these social media data. How to integrate multiple heterogeneous information and establish user profiles from multiple perspectives plays an important role in providing personalized services, marketing, and recommendation systems. In this paper, we propose Multi-source & Multi-task Learning for User Profiles in Social Network which integrates multiple social data sources and contains a multi-task learning framework to simultaneously predict various attributes of a user. Firstly, we design their own feature extraction models for multiple heterogeneous data sources. Secondly, we design a shared layer to fuse multiple heterogeneous data sources as general shared representation for multi-task learning. Thirdly, we design each task’s own unique presentation layer for discriminant output of specific-task. Finally, we design a weighted loss function to improve the learning efficiency and prediction accuracy of each task. Our experimental results on more than 5000 Sina Weibo users demonstrate that our approach outperforms state-of-the-art baselines for inferring gender, age and region of social media users.Keywords
Cite This Article
Citations
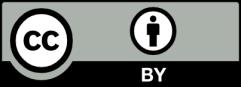
This work is licensed under a Creative Commons Attribution 4.0 International License , which permits unrestricted use, distribution, and reproduction in any medium, provided the original work is properly cited.