Open Access
ARTICLE
Genetic-Frog-Leaping Algorithm for Text Document Clustering
1 Computer Science Department, College of Computer and Information Sciences, King Saud University, Riyadh, Saudi Arabia.
* Corresponding Author: Lubna Alhenaki. Email: lubna.henaki@gmail.com.
Computers, Materials & Continua 2019, 61(3), 1045-1074. https://doi.org/10.32604/cmc.2019.08355
Abstract
In recent years, the volume of information in digital form has increased tremendously owing to the increased popularity of the World Wide Web. As a result, the use of techniques for extracting useful information from large collections of data, and particularly documents, has become more necessary and challenging. Text clustering is such a technique; it consists in dividing a set of text documents into clusters (groups), so that documents within the same cluster are closely related, whereas documents in different clusters are as different as possible. Clustering depends on measuring the content (i.e., words) of a document in terms of relevance. Nevertheless, as documents usually contain a large number of words, some of them may be irrelevant to the topic under consideration or redundant. This can confuse and complicate the clustering process and make it less accurate. Accordingly, feature selection methods have been employed to reduce data dimensionality by selecting the most relevant features. In this study, we developed a text document clustering optimization model using a novel genetic frog-leaping algorithm that efficiently clusters text documents based on selected features. The proposed approach is based on two metaheuristic algorithms: a genetic algorithm (GA) and a shuffled frog-leaping algorithm (SFLA). The GA performs feature selection, and the SFLA performs clustering. To evaluate its effectiveness, the proposed approach was tested on a well-known text document dataset: the “20Newsgroup” dataset from the University of California Irvine Machine Learning Repository. Overall, after multiple experiments were compared and analyzed, it was demonstrated that using the proposed algorithm on the 20Newsgroup dataset greatly facilitated text document clustering, compared with classical K-means clustering. Nevertheless, this improvement requires longer computational time.Keywords
Cite This Article
Citations
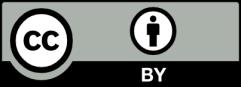