Open Access
ARTICLE
SVM Model Selection Using PSO for Learning Handwritten Arabic Characters
1 Département Informatique Université des Sciences et de la Technologie d’Oran Mohamed Boudiaf USTOMB, BP 1505 El M’naoeur, 31000, Oran, Algérie.
* Corresponding Author: Mamouni El Mamoun. Email: elmamoun.mamouni@univ-usto.dz.
Computers, Materials & Continua 2019, 61(3), 995-1008. https://doi.org/10.32604/cmc.2019.08081
Abstract
Using Support Vector Machine (SVM) requires the selection of several parameters such as multi-class strategy type (one-against-all or one-against-one), the regularization parameter C, kernel function and their parameters. The choice of these parameters has a great influence on the performance of the final classifier. This paper considers the grid search method and the particle swarm optimization (PSO) technique that have allowed to quickly select and scan a large space of SVM parameters. A comparative study of the SVM models is also presented to examine the convergence speed and the results of each model. SVM is applied to handwritten Arabic characters learning, with a database containing 4840 Arabic characters in their different positions (isolated, beginning, middle and end). Some very promising results have been achieved.Keywords
Cite This Article
Citations
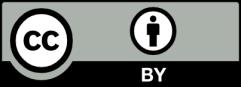
This work is licensed under a Creative Commons Attribution 4.0 International License , which permits unrestricted use, distribution, and reproduction in any medium, provided the original work is properly cited.