Open Access
ARTICLE
Long Short Term Memory Networks Based Anomaly Detection for KPIs
School of Computer Science and Technology, Harbin Institute of Technology, Harbin, 150000, China.
Institute of Computer Application, China Academy of Engineer Physics, Mianyang, 621900, China.
Department of Software, Sejong University, Seoul, Korea.
Cyberspace Institute of Advanced Technology, Guangzhou University, Guangzhou, 510006, China.
*Corresponding Authors: Fanzhi Meng. Email: " />; Mohan Li. Email:
" />
Computers, Materials & Continua 2019, 61(2), 829-847. https://doi.org/10.32604/cmc.2019.06115
Abstract
In real-world many internet-based service companies need to closely monitor large amounts of data in order to ensure stable operation of their business. However, anomaly detection for these data with various patterns and data quality has been a great challenge, especially without labels. In this paper, we adopt an anomaly detection algorithm based on Long Short-Term Memory (LSTM) Network in terms of reconstructing KPIs and predicting KPIs. They use the reconstruction error and prediction error respectively as the criteria for judging anomalies, and we test our method with real data from a company in the insurance industry and achieved good performance.Keywords
Cite This Article
Citations
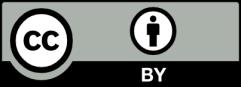
This work is licensed under a Creative Commons Attribution 4.0 International License , which permits unrestricted use, distribution, and reproduction in any medium, provided the original work is properly cited.