Open Access
ARTICLE
An Improved End-to-End Memory Network for QA Tasks
School of Computer and Communication Engineering, University of Science and Technology Beijing, Beijing, 100083, China.
Beijing Key Laboratory of Knowledge Engineering for Materials Science, Beijing, 100083, China.
Amphenol Assemble Tech, Houston, TX 77070, US.
https://github.com/zhenqicool/An-Improved-End-To-End-Memory-Network-For-QA-Tasks-in-PyTorch.
* Corresponding Author: Yonghong Xie. Email: .
Computers, Materials & Continua 2019, 60(3), 1283-1295. https://doi.org/10.32604/cmc.2019.07722
Abstract
At present, End-to-End trainable Memory Networks (MemN2N) has proven to be promising in many deep learning fields, especially on simple natural language-based reasoning question and answer (QA) tasks. However, when solving some subtasks such as basic induction, path finding or time reasoning tasks, it remains challenging because of limited ability to learn useful information between memory and query. In this paper, we propose a novel gated linear units (GLU) and local-attention based end-to-end memory networks (MemN2N-GL) motivated by the success of attention mechanism theory in the field of neural machine translation, it shows an improved possibility to develop the ability of capturing complex memory-query relations and works better on some subtasks. It is an improved end-to-end memory network for QA tasks. We demonstrate the effectiveness of these approaches on the 20 bAbI dataset which includes 20 challenging tasks, without the use of any domain knowledge. Our project is open source on github4.Keywords
Cite This Article
Citations
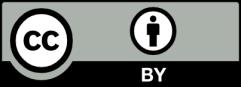
This work is licensed under a Creative Commons Attribution 4.0 International License , which permits unrestricted use, distribution, and reproduction in any medium, provided the original work is properly cited.