Open Access
ARTICLE
Distant Supervised Relation Extraction with Cost-Sensitive Loss
Hunan Provincial Key Laboratory of Intelligent Processing of Big Data on Transportation, School of Computer & Communication Engineering, Changsha University of Science & Technology, Changsha, 410004, China.
School of Computer & Communication Engineering, Changsha University of Science & Technology, Changsha, 410004, China.
School of Computing Science and Engineering, Vellore Institute of Technology (VIT), Tamil Nadu 637212, India.
* Corresponding Author: Jin Wang. Email: .
Computers, Materials & Continua 2019, 60(3), 1251-1261. https://doi.org/10.32604/cmc.2019.06100
Abstract
Recently, many researchers have concentrated on distant supervision relation extraction (DSRE). DSRE has solved the problem of the lack of data for supervised learning, however, the data automatically labeled by DSRE has a serious problem, which is class imbalance. The data from the majority class obviously dominates the dataset, in this case, most neural network classifiers will have a strong bias towards the majority class, so they cannot correctly classify the minority class. Studies have shown that the degree of separability between classes greatly determines the performance of imbalanced data. Therefore, in this paper we propose a novel model, which combines class-to-class separability and cost-sensitive learning to adjust the maximum reachable cost of misclassification, thus improving the performance of imbalanced data sets under distant supervision. Experiments have shown that our method is more effective for DSRE than baseline methods.Keywords
Cite This Article
Citations
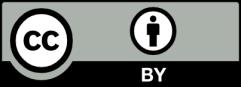
This work is licensed under a Creative Commons Attribution 4.0 International License , which permits unrestricted use, distribution, and reproduction in any medium, provided the original work is properly cited.