Open Access
ARTICLE
Few-Shot Learning with Generative Adversarial Networks Based on WOA13 Data
College of Computer Science and Technology, Jilin University, Changchun, 130012, China.
Key Laboratory of Symbolic Computation and Knowledge Engineering, Jilin University, Changchun, 130012, China.
Department of Engineering Mechanics, State Marine Technical University of St. Petersburg, St. Petersburg, 190008, Russia.
* Corresponding Author: Yu Jiang. Email: .
Computers, Materials & Continua 2019, 60(3), 1073-1085. https://doi.org/10.32604/cmc.2019.05929
Abstract
In recent years, extreme weather events accompanying the global warming have occurred frequently, which brought significant impact on national economic and social development. The ocean is an important member of the climate system and plays an important role in the occurrence of climate anomalies. With continuous improvement of sensor technology, we use sensors to acquire the ocean data for the study on resource detection and disaster prevention, etc. However, the data acquired by the sensor is not enough to be used directly by researchers, so we use the Generative Adversarial Network (GAN) to enhance the ocean data. We use GAN to process WOA13 dataset and use ResNet to determine if there is a thermocline layer in a sea area. We compare the classification results of the enhanced datasets of different orders of magnitude with the classification results of the original datasets. The experimental result shows that the dataset processed by GAN has a higher accuracy. GAN has a certain enhancement effect to marine data. Gan increased the accuracy of the WOA dataset from 0.91 to 0.93. At the same time, the experimental results also show that too much data cannot continue to enhance the accuracy of WOA in ResNet.Keywords
Cite This Article
Citations
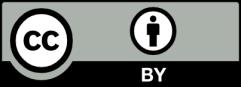