Open Access
ARTICLE
Drug Side-Effect Prediction Using Heterogeneous Features and Bipartite Local Models
Faculty of Engineering and Information Technology, University of Technology Sydney, Sydney, 2205, Australia.
College of Computer, National University of Defense Technology, Changsha, 410073, China.
* Corresponding Author: Wentao Zhao. Email: .
Computers, Materials & Continua 2019, 60(2), 481-496. https://doi.org/10.32604/cmc.2019.05536
Abstract
Drug side-effects impose massive costs on society, leading to almost one-third drug failure in the drug discovery process. Therefore, early identification of potential side-effects becomes vital to avoid risks and reduce costs. Existing computational methods employ few drug features and predict drug side-effects from either drug side or side-effect side separately. In this work, we explore to predict drug side-effects by combining heterogeneous drug features and employing the bipartite local models (BLMs) which fuse predictions from both the drug side and side-effect side. Specifically, we integrate drug chemical structures, drug interacted proteins and drug associated genes into a unified framework to measure the comprehensive similarity between drugs first. Then, high-quality and balanced training samples are selected for individual drugs and individual side-effects using the designed balanced sample selection framework, based on drug comprehensive similarities and side-effect cosine similarities respectively. Trained with corresponding training samples, BLMs first predict drugs associated with a given side-effect, then predict side-effects for a given drug. This produces two independent predictions for each putative drug-side-effect association which are further combined to give a definitive prediction. The performance of the proposed method was evaluated on side-effect prediction for 901 drugs from DrugBank. Particularly, we performed 5-fold cross-validation experiments on the 742 characterized drugs and independent testing experiment on the 159 uncharacterized drugs. The simulative predictions show that the side-effect prediction performance is significantly improved owing to the integration of information from drug chemical, biological and genomic spaces, the proposed sample selection framework, and the implemented BLMs.Keywords
Cite This Article
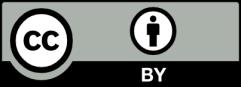