Open Access
ARTICLE
Robust Re-Weighted Multi-View Feature Selection
* Corresponding Author: Ping Zhong. Email: .
Computers, Materials & Continua 2019, 60(2), 741-756. https://doi.org/10.32604/cmc.2019.05611
Abstract
In practical application, many objects are described by multi-view features because multiple views can provide a more informative representation than the single view. When dealing with the multi-view data, the high dimensionality is often an obstacle as it can bring the expensive time consumption and an increased chance of over-fitting. So how to identify the relevant views and features is an important issue. The matrix-based multi-view feature selection that can integrate multiple views to select relevant feature subset has aroused widely concern in recent years. The existing supervised multi-view feature selection methods usually concatenate all views into the long vectors to design the models. However, this concatenation has no physical meaning and indicates that different views play the similar roles for a specific task. In this paper, we propose a robust re-weighted multi-view feature selection method by constructing the penalty term based on the low-dimensional subspaces of each view through the least-absolute criterion. The proposed model can fully consider the complementary property of multiple views and the specificity of each view. It can not only induce robustness to mitigate the impacts of outliers, but also learn the corresponding weights adaptively for different views without any presetting parameter. In the process of optimization, the proposed model can be splitted to several small scale sub-problems. An iterative algorithm based on the iteratively re-weighted least squares is proposed to efficiently solve these sub-problems. Furthermore, the convergence of the iterative algorithm is theoretical analyzed. Extensive comparable experiments with several state-of-the-art feature selection methods verify the effectiveness of the proposed method.Keywords
Cite This Article
Citations
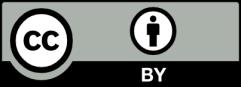
This work is licensed under a Creative Commons Attribution 4.0 International License , which permits unrestricted use, distribution, and reproduction in any medium, provided the original work is properly cited.