Open Access
ARTICLE
Collaborative Filtering Recommendation Algorithm Based on Multi-Relationship Social Network
School of Data Science and Software Engineering, Qingdao University, Qingdao, 266071, China.
College of Information Engineering, Sanming University, Sanming, 365004, China.
Department of Information Engineering, Fujian College of Water Conservancy and Electric Power, Yongan, 366000, China.
School of Internet of Things and Technology, Wuxi Vocational Institute of Commerce, Wuxi, 214153, China.
College of Information Engineering, Shandong Yingcai University, Jinan, 250104, China.
School of Electronic and Information, Shandong Modern University, Jinan, 250104, China.
School of Computer Science, University College Dublin, Dublin, Ireland.
* Corresponding Author: Sheng Bin. Email: .
Computers, Materials & Continua 2019, 60(2), 659-674. https://doi.org/10.32604/cmc.2019.05858
Abstract
Recommendation system is one of the most common applications in the field of big data. The traditional collaborative filtering recommendation algorithm is directly based on user-item rating matrix. However, when there are huge amounts of user and commodities data, the efficiency of the algorithm will be significantly reduced. Aiming at the problem, a collaborative filtering recommendation algorithm based on multi-relational social networks is proposed. The algorithm divides the multi-relational social networks based on the multi-subnet complex network model into communities by using information dissemination method, which divides the users with similar degree into a community. Then the user-item rating matrix is constructed by choosing the k-nearest neighbor set of users within the community, in this case, the collaborative filtering algorithm is used for recommendation. Thus, the execution efficiency of the algorithm is improved without reducing the accuracy of recommendation.Keywords
Cite This Article
Citations
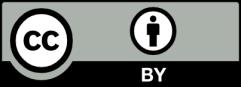
This work is licensed under a Creative Commons Attribution 4.0 International License , which permits unrestricted use, distribution, and reproduction in any medium, provided the original work is properly cited.