Open Access
ARTICLE
Traffic Sign Recognition Method Integrating Multi-Layer Features and Kernel Extreme Learning Machine Classifier
School of Information and Control, Nanjing University of Information Science & Technology, Nanjing, 210044, China.
School of Computer and Software, Nanjing University of Information Science & Technology, Nanjing, 210044, China.
Jiangsu Collaborative Innovation Center of Atmospheric Environment and Equipment Technology, Nanjing, 210044, China.
Department of Civil and Environmental Engineering, Rensselaer Polytechnic Institute, New York, 12180, USA.
* Corresponding Author: Wei Sun. Email: .
Computers, Materials & Continua 2019, 60(1), 147-161. https://doi.org/10.32604/cmc.2019.03581
Abstract
Traffic sign recognition (TSR), as a critical task to automated driving and driver assistance systems, is challenging due to the color fading, motion blur, and occlusion. Traditional methods based on convolutional neural network (CNN) only use an end-layer feature as the input to TSR that requires massive data for network training. The computation-intensive network training process results in an inaccurate or delayed classification. Thereby, the current state-of-the-art methods have limited applications. This paper proposes a new TSR method integrating multi-layer feature and kernel extreme learning machine (ELM) classifier. The proposed method applies CNN to extract the multi-layer features of traffic signs, which can present sufficient details and semantically abstract information of multi-layer feature maps. The extraction of multi-scale features of traffic signs is effective against object scale variation by applying a new multi-scale pooling operation. Further, the extracted features are combined into a multi-scale multi-attribute vector, which can enhance the feature presentation ability for TSR. To efficiently handle nonlinear sampling problems in TSR, the kernel ELM classifier is adopted for efficient TSR. The kernel ELM has a more powerful function approximation capability, which can achieve an optimal and generalized solution for multiclass TSR. Experimental results demonstrate that the proposed method can improve the recognition accuracy, efficiency, and adaptivity to complex travel environments in TSR.Keywords
Cite This Article
Citations
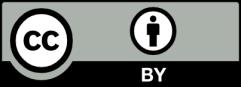
This work is licensed under a Creative Commons Attribution 4.0 International License , which permits unrestricted use, distribution, and reproduction in any medium, provided the original work is properly cited.