Open Access
ARTICLE
DPIF: A Framework for Distinguishing Unintentional Quality Problems From Potential Shilling Attacks
Cyberspace Institute of Advanced Technology, Guangzhou University, Guangzhou, 510006, China.
School of Software, University of Technology Sydney, Sydney, Australia.
* Corresponding Authors: Yanbin Sun. Email: ;
Yuhang Wang. Email: .
Computers, Materials & Continua 2019, 59(1), 331-344. https://doi.org/10.32604/cmc.2019.05379
Abstract
Maliciously manufactured user profiles are often generated in batch for shilling attacks. These profiles may bring in a lot of quality problems but not worthy to be repaired. Since repairing data always be expensive, we need to scrutinize the data and pick out the data that really deserves to be repaired. In this paper, we focus on how to distinguish the unintentional data quality problems from the batch generated fake users for shilling attacks. A two-steps framework named DPIF is proposed for the distinguishment. Based on the framework, the metrics of homology and suspicious degree are proposed. The homology can be used to represent both the similarities of text and the data quality problems contained by different profiles. The suspicious degree can be used to identify potential attacks. The experiments on real-life data verified that the proposed framework and the corresponding metrics are effective.Keywords
Cite This Article
Citations
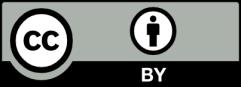
This work is licensed under a Creative Commons Attribution 4.0 International License , which permits unrestricted use, distribution, and reproduction in any medium, provided the original work is properly cited.