Open Access
ARTICLE
Deep Q-Learning Based Computation Offloading Strategy for Mobile Edge Computing
Beijing Key Laboratory of Work Safety Intelligent Monitoring, School of Electronic Engineering, Beijing University of Posts and Telecommunications, Beijing, 100876, China.
Department of Systems and Computer Engineering, Carleton University, Ottawa, ON K1S 5B6, Canada.
* Corresponding Author: Yifei Wei. Email: .
Computers, Materials & Continua 2019, 59(1), 89-104. https://doi.org/10.32604/cmc.2019.04836
Abstract
To reduce the transmission latency and mitigate the backhaul burden of the centralized cloud-based network services, the mobile edge computing (MEC) has been drawing increased attention from both industry and academia recently. This paper focuses on mobile users’ computation offloading problem in wireless cellular networks with mobile edge computing for the purpose of optimizing the computation offloading decision making policy. Since wireless network states and computing requests have stochastic properties and the environment’s dynamics are unknown, we use the model-free reinforcement learning (RL) framework to formulate and tackle the computation offloading problem. Each mobile user learns through interactions with the environment and the estimate of its performance in the form of value function, then it chooses the overhead-aware optimal computation offloading action (local computing or edge computing) based on its state. The state spaces are high-dimensional in our work and value function is unrealistic to estimate. Consequently, we use deep reinforcement learning algorithm, which combines RL method Q-learning with the deep neural network (DNN) to approximate the value functions for complicated control applications, and the optimal policy will be obtained when the value function reaches convergence. Simulation results showed that the effectiveness of the proposed method in comparison with baseline methods in terms of total overheads of all mobile users.Keywords
Cite This Article
Citations
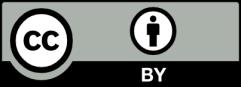
This work is licensed under a Creative Commons Attribution 4.0 International License , which permits unrestricted use, distribution, and reproduction in any medium, provided the original work is properly cited.