Open Access
ARTICLE
The Prediction of Self-Healing Capacity of Bacteria-Based Concrete Using Machine Learning Approaches
Division of Computational Mechanics, Ton Duc Thang University, Ho Chi Minh City, Vietnam.
Faculty of Civil Engineering, Ton Duc Thang University, Ho Chi Minh City, Vietnam.
College of Materials Science and Engineering, Chongqing University, Chongqing, 400045, China.
Institute of Continuum Mechanics, Leibniz University Hannover, Hannover, 30167, Germany.
* Corresponding Author: Xiaoying Zhuang. Email: .
Computers, Materials & Continua 2019, 59(1), 57-77. https://doi.org/10.32604/cmc.2019.04589
Abstract
Advances in machine learning (ML) methods are important in industrial engineering and attract great attention in recent years. However, a comprehensive comparative study of the most advanced ML algorithms is lacking. Six integrated ML approaches for the crack repairing capacity of the bacteria-based self-healing concrete are proposed and compared. Six ML algorithms, including the Support Vector Regression (SVR), Decision Tree Regression (DTR), Gradient Boosting Regression (GBR), Artificial Neural Network (ANN), Bayesian Ridge Regression (BRR) and Kernel Ridge Regression (KRR), are adopted for the relationship modeling to predict crack closure percentage (CCP). Particle Swarm Optimization (PSO) is used for the hyper-parameters tuning. The importance of parameters is analyzed. It is demonstrated that integrated ML approaches have great potential to predict the CCP, and PSO is efficient in the hyper-parameter tuning. This research provides useful information for the design of the bacteria-based self-healing concrete and can contribute to the design in the rest of industrial engineering.Keywords
Cite This Article
Citations
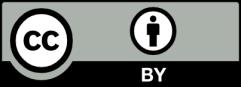