Open Access
ARTICLE
R2N: A Novel Deep Learning Architecture for Rain Removal from Single Image
School of Electronic and Information Engineering, Nanjing University of Information Science and Technology, Nanjing Jiangsu, 210044, China.
Jiangsu Collaborative Innovation Center of Atmospheric Environment and Equipment Technology, Nanjing Jiangsu, 210044, China.
School of Computing, Edinburgh Napier University, Edinburgh, EH10 5DT, UK.
* Corresponding Author: Yecai Guo. Email: .
Computers, Materials & Continua 2019, 58(3), 829-843. https://doi.org/10.32604/cmc.2019.03729
Abstract
Visual degradation of captured images caused by rainy streaks under rainy weather can adversely affect the performance of many open-air vision systems. Hence, it is necessary to address the problem of eliminating rain streaks from the individual rainy image. In this work, a deep convolution neural network (CNN) based method is introduced, called Rain-Removal Net (R2N), to solve the single image de-raining issue. Firstly, we decomposed the rainy image into its high-frequency detail layer and low-frequency base layer. Then, we used the high-frequency detail layer to input the carefully designed CNN architecture to learn the mapping between it and its corresponding de-rained high-frequency detail layer. The CNN architecture consists of four convolution layers and four deconvolution layers, as well as three skip connections. The experiments on synthetic and real-world rainy images show that the performance of our architecture outperforms the compared state-of-the-art de-raining models with respects to the quality of de-rained images and computing efficiency.Keywords
Cite This Article
Citations
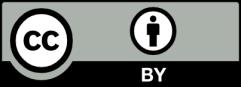