Open Access
ARTICLE
An Entity-Association-Based Matrix Factorization Recommendation Algorithm
School of Electronic Information and Electrical Engineering, Shanghai Jiao Tong University, Shanghai, 200240, China.
* Corresponding Author: Meng Kui. Email: .
Computers, Materials & Continua 2019, 58(1), 101-120. https://doi.org/10.32604/cmc.2019.03898
Abstract
Collaborative filtering is the most popular approach when building recommender systems, but the large scale and sparse data of the user-item matrix seriously affect the recommendation results. Recent research shows the user’s social relations information can improve the quality of recommendation. However, most of the current social recommendation algorithms only consider the user's direct social relations, while ignoring potential users’ interest preference and group clustering information. Moreover, project attribute is also important in item rating. We propose a recommendation algorithm which using matrix factorization technology to fuse user information and project information together. We first detect the community structure using overlapping community discovery algorithm, and mine the clustering information of user interest preference by a fuzzy clustering algorithm based on the project category information. On the other hand, we use project-category attribution matrix and user-project score matrix to get project comprehensive similarity and compute project feature matrix based on Entity Relation Decomposition. Fusing the user clustering information and project information together, we get Entity-Association-based Matrix Factorization (EAMF) model which can be used to predict user ratings. The proposed algorithm is compared with other algorithms on the Yelp dataset. Experimental studies show that the proposed algorithm leads to a substantial increase in recommendation accuracy on Yelp data set.Keywords
Cite This Article
Citations
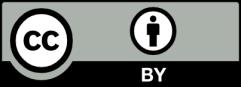
This work is licensed under a Creative Commons Attribution 4.0 International License , which permits unrestricted use, distribution, and reproduction in any medium, provided the original work is properly cited.