Open Access
ARTICLE
Using Imbalanced Triangle Synthetic Data for Machine Learning Anomaly Detection
College of Computer, National University of Defense Technology, Changsha, 410073, China.
Normal College of Jishou University, Jishou, 416000, China.
School of Information Science and Engineering, Central South University, Changsha, 410083, China .
Innovation Center, China Academy of Electronics and Information Technology, Beijing, 100041, China .
Faculty of Information Technology, Macau University of Science and Technology, 519020, MACAU.
* Corresponding Author: Zhiping Cai. Email: .
Computers, Materials & Continua 2019, 58(1), 15-26. https://doi.org/10.32604/cmc.2019.03708
Abstract
The extreme imbalanced data problem is the core issue in anomaly detection. The amount of abnormal data is so small that we cannot get adequate information to analyze it. The mainstream methods focus on taking fully advantages of the normal data, of which the discrimination method is that the data not belonging to normal data distribution is the anomaly. From the view of data science, we concentrate on the abnormal data and generate artificial abnormal samples by machine learning method. In this kind of technologies, Synthetic Minority Over-sampling Technique and its improved algorithms are representative milestones, which generate synthetic examples randomly in selected line segments. In our work, we break the limitation of line segment and propose an Imbalanced Triangle Synthetic Data method. In theory, our method covers a wider range. In experiment with real world data, our method performs better than the SMOTE and its meliorations.Keywords
Cite This Article
Citations
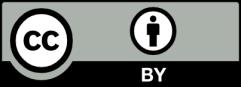