Open Access
ARTICLE
Research on Hybrid Model of Garlic Short-term Price Forecasting based on Big Data
College of information science and engineering, Shandong Agricultural University, Tai’an, 271000, China.
College of Information Engineering, Qingdao Binhai University, Qingdao, 266555, China.
School of Computer Science, University College Dublin (UCD), Belfield, Dublin 4, Ireland.
* Corresponding Author: Pingzeng Liu. Email: .
Computers, Materials & Continua 2018, 57(2), 283-296. https://doi.org/10.32604/cmc.2018.03791
Abstract
Garlic prices fluctuate dramatically in recent years and it is very difficult to predict garlic prices. The autoregressive integrated moving average (ARIMA) model is currently the most important method for predicting garlic prices. However, the ARIMA model can only predict the linear part of the garlic prices, and cannot predict its nonlinear part. Therefore, it is urgent to adopt a method to analyze the nonlinear characteristics of garlic prices. After comparing the advantages and disadvantages of several major prediction models which used to forecast nonlinear time series, using support vector machine (SVM) model to predict the nonlinear part of garlic prices and establish ARIMA-SVM hybrid forecast model to predict garlic prices. The monthly average price data of garlic in 2010-2017 was used to test the effect of ARIMA model, SVM model and ARIMA-SVM model. The experimental results show that: (1) Garlic price is affected by many factors but the most is the supply and demand relationship; (2) The SVM model has a good effect in dealing with the nonlinear relationship of garlic prices; (3) The ARIMA-SVM hybrid model is better than the single ARIMA model and SVM model on the accuracy of garlic price prediction, it can be used as an effective method to predict the short-term price of garlic.Keywords
Cite This Article
Citations
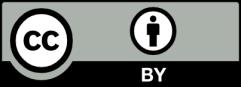