Open Access
ARTICLE
Snow Cover Mapping for Mountainous Areas by Fusion of MODIS L1B and Geographic Data Based on Stacked Denoising Auto-Encoders
School of Atmospheric Science, Nanjing University of Information Science and Technology, Nanjing, 210044, China.
School of Information and Control, Nanjing University of Information Science and Technology, Nanjing, 210044, China.
School of Atmospheric Physics, Nanjing University of Information Science and Technology, Nanjing, 210044, China.
School of Computer and Software, Nanjing University of Information Science and Technology, Nanjing, 210044, China.
Department of Computer Engineering, Chosun University, Gwangju, 501-759, South Korea.
* Corresponding Author: Yonghong Zhang. Email: .
Computers, Materials & Continua 2018, 57(1), 49-68. https://doi.org/10.32604/cmc.2018.02376
Abstract
Snow cover plays an important role in meteorological and hydrological researches. However, the accuracies of currently available snow cover products are significantly lower in mountainous areas than in plains, due to the serious snow/cloud confusion problem caused by high altitude and complex topography. Aiming at this problem, an improved snow cover mapping approach for mountainous areas was proposed and applied in Qinghai-Tibetan Plateau. In this work, a deep learning framework named Stacked Denoising Auto-Encoders (SDAE) was employed to fuse the MODIS multispectral images and various geographic datasets, which are then classified into three categories: Snow, cloud and snow-free land. Moreover, two independent SDAE models were trained for snow mapping in snow and snow-free seasons respectively in response to the seasonal variations of meteorological conditions. The proposed approach was verified using in-situ snow depth records, and compared to the most widely used snow products MOD10A1 and MYD10A1. The comparison results show that our method got the best performance: Overall accuracy of 98.95% and F-measure of 73.84%. The results indicated that our method can effectively improve the snow recognition accuracy, and it can be further extended to other multi-source remote sensing image classification issues.Keywords
Cite This Article
Citations
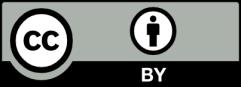
This work is licensed under a Creative Commons Attribution 4.0 International License , which permits unrestricted use, distribution, and reproduction in any medium, provided the original work is properly cited.