Open Access
ARTICLE
Analyzing Cross-domain Transportation Big Data of New York City with Semi-supervised and Active Learning
Computer Science Department, New York University, New York, NY 10012, USA.
* Corresponding Author: Huiyu Sun. Email: .
Computers, Materials & Continua 2018, 57(1), 1-9. https://doi.org/10.32604/cmc.2018.03684
Abstract
The majority of big data analytics applied to transportation datasets suffer from being too domain-specific, that is, they draw conclusions for a dataset based on analytics on the same dataset. This makes models trained from one domain (e.g. taxi data) applies badly to a different domain (e.g. Uber data). To achieve accurate analyses on a new domain, substantial amounts of data must be available, which limits practical applications. To remedy this, we propose to use semi-supervised and active learning of big data to accomplish the domain adaptation task: Selectively choosing a small amount of datapoints from a new domain while achieving comparable performances to using all the datapoints. We choose the New York City (NYC) transportation data of taxi and Uber as our dataset, simulating different domains with 90% as the source data domain for training and the remaining 10% as the target data domain for evaluation. We propose semi-supervised and active learning strategies and apply it to the source domain for selecting datapoints. Experimental results show that our adaptation achieves a comparable performance of using all datapoints while using only a fraction of them, substantially reducing the amount of data required. Our approach has two major advantages: It can make accurate analytics and predictions when big datasets are not available, and even if big datasets are available, our approach chooses the most informative datapoints out of the dataset, making the process much more efficient without having to process huge amounts of data.Keywords
Cite This Article
Citations
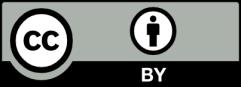
This work is licensed under a Creative Commons Attribution 4.0 International License , which permits unrestricted use, distribution, and reproduction in any medium, provided the original work is properly cited.