Open Access
ARTICLE
Fast Near-duplicate Image Detection in Riemannian Space by A Novel Hashing Scheme
Guangzhou University, Guangzhou High Education Mega Center, Guangzhou, 510006, China.
Illinois Institute of Technology, 3440 South Dearborn, Suit 100, Chicago, IL, 60616, USA.
* Corresponding Author: Ligang Zheng. Email: .
Computers, Materials & Continua 2018, 56(3), 529-539. https://doi.org/10.3970/cmc.2018.03780
Abstract
There is a steep increase in data encoded as symmetric positive definite (SPD) matrix in the past decade. The set of SPD matrices forms a Riemannian manifold that constitutes a half convex cone in the vector space of matrices, which we sometimes call SPD manifold. One of the fundamental problems in the application of SPD manifold is to find the nearest neighbor of a queried SPD matrix. Hashing is a popular method that can be used for the nearest neighbor search. However, hashing cannot be directly applied to SPD manifold due to its non-Euclidean intrinsic geometry. Inspired by the idea of kernel trick, a new hashing scheme for SPD manifold by random projection and quantization in expanded data space is proposed in this paper. Experimental results in large scale near-duplicate image detection show the effectiveness and efficiency of the proposed methodKeywords
Cite This Article
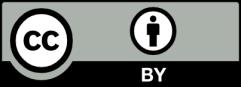