Open Access
ARTICLE
Semi-Supervised Learning with Generative Adversarial Networks on Digital Signal Modulation Classification
College of Information and Communication Engineering, Harbin Engineering University, Harbin 150001, China.
School of Computer & Communication Engineering, Changsha University of Science & Technology, Changsha 410114, China.
School of Information Engineering, Yangzhou University, Yangzhou 225009, China.
Department of Energy Grid, Sangmyung University, Seoul, Korea.
* Corresponding author: Jin Wang. Email: .
Computers, Materials & Continua 2018, 55(2), 243-254. https://doi.org/10.3970/cmc.2018.01755
Abstract
Deep Learning (DL) is such a powerful tool that we have seen tremendous success in areas such as Computer Vision, Speech Recognition, and Natural Language Pro-cessing. Since Automated Modulation Classification (AMC) is an important part in Cognitive Radio Networks, we try to explore its potential in solving signal modula-tion recognition problem. It cannot be overlooked that DL model is a complex mod-el, thus making them prone to over-fitting. DL model requires many training data to combat with over-fitting, but adding high quality labels to training data manually is not always cheap and accessible, especially in real-time system, which may counter unprecedented data in dataset. Semi-supervised Learning is a way to exploit unla-beled data effectively to reduce over-fitting in DL. In this paper, we extend Genera-tive Adversarial Networks (GANs) to the semi-supervised learning will show it is a method can be used to create a more data-efficient classifier.Keywords
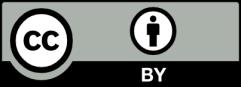