Open Access
ARTICLE
Fingerprint Liveness Detection from Different Fingerprint Materials Using Convolutional Neural Network and Principal Component Analysis
School of Computer and Software, Nanjing University of Information Science & Technology, Ning Liu Road, No. 219, Nanjing, China, 210044
Jiangsu Engineering Center of Network Monitoring, Ning Liu Road, No. 219, Nanjing, China, 210044
Department of Electrical and Computer Engineering, University of Windsor, 401 Sunset Avenue, Windsor, ON, Canada N9B 3P4
School of Computer Science, Guangzhou University, Yudongxi Road 36, Tianhe District, Guangzhou, China, 510500.
The corresponding author: Jin Li, E-mail: jinli71@gmail.com
Computers, Materials & Continua 2017, 53(4), 357-372. https://doi.org/10.3970/cmc.2017.053.357
Abstract
Fingerprint-spoofing attack often occurs when imposters gain access illegally by using artificial fingerprints, which are made of common fingerprint materials, such as silicon, latex, etc. Thus, to protect our privacy, many fingerprint liveness detection methods are put forward to discriminate fake or true fingerprint. Current work on liveness detection for fingerprint images is focused on the construction of complex handcrafted features, but these methods normally destroy or lose spatial information between pixels. Different from existing methods, convolutional neural network (CNN) can generate high-level semantic representations by learning and concatenating low-level edge and shape features from a large amount of labeled data. Thus, CNN is explored to solve the above problem and discriminate true fingerprints from fake ones in this paper. To reduce the redundant information and extract the most distinct features, ROI and PCA operations are performed for learned features of convolutional layer or pooling layer. After that, the extracted features are fed into SVM classifier. Experimental results based on the LivDet 2013 and the LivDet 2011 datasets, which are captured by using different fingerprint materials, indicate that the classification performance of our proposed method is both efficient and convenient compared with the other previous methods.Keywords
Cite This Article
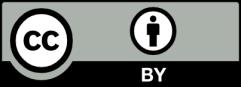
This work is licensed under a Creative Commons Attribution 4.0 International License , which permits unrestricted use, distribution, and reproduction in any medium, provided the original work is properly cited.