Open Access
ARTICLE
Dynamic Spatial Focus in Alzheimer’s Disease Diagnosis via Multiple CNN Architectures and Dynamic GradNet
Department of Computer Science, College of Computers and Information Technology, Taif University, Taif, 21944, Saudi Arabia
* Corresponding Author: Jasem Almotiri. Email:
(This article belongs to the Special Issue: Cutting-Edge Machine Learning and AI Innovations in Medical Imaging Diagnosis)
Computers, Materials & Continua 2025, 83(2), 2109-2142. https://doi.org/10.32604/cmc.2025.062923
Received 31 December 2024; Accepted 17 March 2025; Issue published 16 April 2025
Abstract
The evolving field of Alzheimer’s disease (AD) diagnosis has greatly benefited from deep learning models for analyzing brain magnetic resonance (MR) images. This study introduces Dynamic GradNet, a novel deep learning model designed to increase diagnostic accuracy and interpretability for multiclass AD classification. Initially, four state-of-the-art convolutional neural network (CNN) architectures, the self-regulated network (RegNet), residual network (ResNet), densely connected convolutional network (DenseNet), and efficient network (EfficientNet), were comprehensively compared via a unified preprocessing pipeline to ensure a fair evaluation. Among these models, EfficientNet consistently demonstrated superior performance in terms of accuracy, precision, recall, and F1 score. As a result, EfficientNet was selected as the foundation for implementing Dynamic GradNet. Dynamic GradNet incorporates gradient weighted class activation mapping (GradCAM) into the training process, facilitating dynamic adjustments that focus on critical brain regions associated with early dementia detection. These adjustments are particularly effective in identifying subtle changes associated with very mild dementia, enabling early diagnosis and intervention. The model was evaluated with the OASIS dataset, which contains greater than 80,000 brain MR images categorized into four distinct stages of AD progression. The proposed model outperformed the baseline architectures, achieving remarkable generalizability across all stages. This finding was especially evident in early-stage dementia detection, where Dynamic GradNet significantly reduced false positives and enhanced classification metrics. These findings highlight the potential of Dynamic GradNet as a robust and scalable approach for AD diagnosis, providing a promising alternative to traditional attention-based models. The model’s ability to dynamically adjust spatial focus offers a powerful tool in artificial intelligence (AI) assisted precision medicine, particularly in the early detection of neurodegenerative diseases.Keywords
Cite This Article
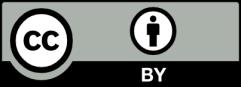
This work is licensed under a Creative Commons Attribution 4.0 International License , which permits unrestricted use, distribution, and reproduction in any medium, provided the original work is properly cited.