Open Access
ARTICLE
A Deep Learning Framework for Arabic Cyberbullying Detection in Social Networks
1 Computer Science Department, Jordan University of Science and Technology, Irbid, 22110, Jordan
2 Department of Information Technology, Faculty of Prince Al-Hussein Bin Abdallah II For Information Technology, The Hashemite University, Zarqa, 13133, Jordan
3 Computer Science Department, Tafila Technical University, Tafila, 66110, Jordan
4 Department of Digital Media Software Engineering, Ferris State University, Big Rapids, MI 49503, USA
5 Department of Telecommunications, University of Ruse “Angel Kanchev”, Ruse, 7017, Bulgaria
* Corresponding Author: Yahya Tashtoush. Email:
(This article belongs to the Special Issue: Applications of Artificial Intelligence for Information Security)
Computers, Materials & Continua 2025, 83(2), 3113-3134. https://doi.org/10.32604/cmc.2025.062724
Received 25 December 2024; Accepted 26 February 2025; Issue published 16 April 2025
Abstract
Social media has emerged as one of the most transformative developments on the internet, revolutionizing the way people communicate and interact. However, alongside its benefits, social media has also given rise to significant challenges, one of the most pressing being cyberbullying. This issue has become a major concern in modern society, particularly due to its profound negative impacts on the mental health and well-being of its victims. In the Arab world, where social media usage is exceptionally high, cyberbullying has become increasingly prevalent, necessitating urgent attention. Early detection of harmful online behavior is critical to fostering safer digital environments and mitigating the adverse effects of cyberbullying. This underscores the importance of developing advanced tools and systems to identify and address such behavior effectively. This paper investigates the development of a robust cyberbullying detection and classification system tailored for Arabic comments on YouTube. The study explores the effectiveness of various deep learning models, including Bi-LSTM (Bidirectional Long Short-Term Memory), LSTM (Long Short-Term Memory), CNN (Convolutional Neural Networks), and a hybrid CNN-LSTM, in classifying Arabic comments into binary classes (bullying or not) and multiclass categories. A comprehensive dataset of 20,000 Arabic YouTube comments was collected, preprocessed, and labeled to support these tasks. The results revealed that the CNN and hybrid CNN-LSTM models achieved the highest accuracy in binary classification, reaching an impressive 91.9%. For multiclass classification, the LSTM and Bi-LSTM models outperformed others, achieving an accuracy of 89.5%. These findings highlight the effectiveness of deep learning approaches in the mitigation of cyberbullying within Arabic online communities.Keywords
Cite This Article
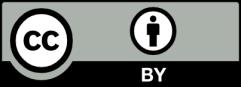
This work is licensed under a Creative Commons Attribution 4.0 International License , which permits unrestricted use, distribution, and reproduction in any medium, provided the original work is properly cited.