Open Access
ARTICLE
ALCTS—An Assistive Learning and Communicative Tool for Speech and Hearing Impaired Students
1 Computer Science Department, College of Computer Engineering and Sciences, Prince Sattam bin Abdulaziz University, Al Kharj, 11942, Saudi Arabia
2 Faculty of Science, Al-Azhar University, Cairo, 12111, Egypt
3 Department of Mathematics, Faculty of Science, Al-Azhar University (Girl’s Branch), Cairo, 12111, Egypt
4 Department of Computer Science and Engineering, Central University of Jammu, Jammu and Kashmir, 181143, India
* Corresponding Author: Shabana Ziyad Puthu Vedu. Email:
Computers, Materials & Continua 2025, 83(2), 2599-2617. https://doi.org/10.32604/cmc.2025.062695
Received 25 December 2024; Accepted 21 February 2025; Issue published 16 April 2025
Abstract
Hearing and Speech impairment can be congenital or acquired. Hearing and speech-impaired students often hesitate to pursue higher education in reputable institutions due to their challenges. However, the development of automated assistive learning tools within the educational field has empowered disabled students to pursue higher education in any field of study. Assistive learning devices enable students to access institutional resources and facilities fully. The proposed assistive learning and communication tool allows hearing and speech-impaired students to interact productively with their teachers and classmates. This tool converts the audio signals into sign language videos for the speech and hearing-impaired to follow and converts the sign language to text format for the teachers to follow. This educational tool for the speech and hearing-impaired is implemented by customized deep learning models such as Convolution neural networks (CNN), Residual neural Networks (ResNet), and stacked Long short-term memory (LSTM) network models. This assistive learning tool is a novel framework that interprets the static and dynamic gesture actions in American Sign Language (ASL). Such communicative tools empower the speech and hearing impaired to communicate effectively in a classroom environment and foster inclusivity. Customized deep learning models were developed and experimentally evaluated with the standard performance metrics. The model exhibits an accuracy of 99.7% for all static gesture classification and 99% for specific vocabulary of gesture action words. This two-way communicative and educational tool encourages social inclusion and a promising career for disabled students.Keywords
Cite This Article
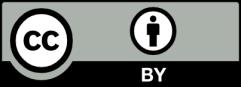
This work is licensed under a Creative Commons Attribution 4.0 International License , which permits unrestricted use, distribution, and reproduction in any medium, provided the original work is properly cited.