Open Access
ARTICLE
UltraSegNet: A Hybrid Deep Learning Framework for Enhanced Breast Cancer Segmentation and Classification on Ultrasound Images
1 Department of Computer Science, Faculty of Prince Al-Hussein Bin Abdallah II for Information Technology, Al Al-Bayt University, Mafraq, 25113, Jordan
2 Medical Engineering Department, Faculty of Engineering, Al-Ahliyya Amman University, Amman, 19328, Jordan
3 Department of Information Technology, College of Computer and Information Sciences, Princess Nourah bint Abdulrahman University, Riyadh, 11671, Saudi Arabia
4 Faculty of Information Technology, Applied Science Private University, Amman, 11931, Jordan
5 Faculty of Computers Science, Misr International University, Cairo, 11800, Egypt
6 Jadara Research Center, Jadara University, Irbid, 21110, Jordan
* Corresponding Authors: Suhaila Abuowaida. Email: ; Deema Mohammed Alsekait. Email:
(This article belongs to the Special Issue: Artificial Intelligence Algorithms and Applications)
Computers, Materials & Continua 2025, 83(2), 3303-3333. https://doi.org/10.32604/cmc.2025.063470
Received 15 January 2025; Accepted 12 March 2025; Issue published 16 April 2025
Abstract
Segmenting a breast ultrasound image is still challenging due to the presence of speckle noise, dependency on the operator, and the variation of image quality. This paper presents the UltraSegNet architecture that addresses these challenges through three key technical innovations: This work adds three things: (1) a changed ResNet-50 backbone with sequential 3 convolutions to keep fine anatomical details that are needed for finding lesion boundaries; (2) a computationally efficient regional attention mechanism that works on high-resolution features without using a transformer’s extra memory; and (3) an adaptive feature fusion strategy that changes local and global features based on how the image is being used. Extensive evaluation on two distinct datasets demonstrates UltraSegNet’s superior performance: On the BUSI dataset, it obtains a precision of 0.915, a recall of 0.908, and an F1 score of 0.911. In the UDAIT dataset, it achieves robust performance across the board, with a precision of 0.901 and recall of 0.894. Importantly, these improvements are achieved at clinically feasible computation times, taking 235 ms per image on standard GPU hardware. Notably, UltraSegNet does amazingly well on difficult small lesions (less than 10 mm), achieving a detection accuracy of 0.891. This is a huge improvement over traditional methods that have a hard time with small-scale features, as standard models can only achieve 0.63–0.71 accuracy. This improvement in small lesion detection is particularly crucial for early-stage breast cancer identification. Results from this work demonstrate that UltraSegNet can be practically deployable in clinical workflows to improve breast cancer screening accuracy.Keywords
Cite This Article
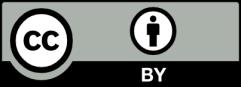
This work is licensed under a Creative Commons Attribution 4.0 International License , which permits unrestricted use, distribution, and reproduction in any medium, provided the original work is properly cited.