Open Access
ARTICLE
A Comparative Study of Optimized-LSTM Models Using Tree-Structured Parzen Estimator for Traffic Flow Forecasting in Intelligent Transportation
1 Department of Electronics, University of Peshawar, Peshawar, 25120, KPK, Pakistan
2 Department of Project Management (L.A.D.L), Higher Polytechnic School (S.G.V), Universidad Europea del Atlántico, Santander, 39011, Spain
3 Faculty of Engineering, Universidad Internacional Iberoamericana, Campeche, 24560, México
4 Faculty of Engineering, Universidade Internacional do Cuanza, Cuito, EN250, Bié, Angola
5 Department of Project Management, Universidad Internacional Iberoamericana, Campeche, 24560, México
6 Department of Project Management, Department of Management Sciences, Universid de La Romana, La Romana, 22000, Dominican Reublic
7 Information Systems Department, College of Computer Science, King Khalid University, Abha, 61421, Saudi Arabia
8 Department of Electrical and Electronic Engineering, College of Engineering & Computer Science, Jazan University, Jazan, 45142, Saudi Arabia
* Corresponding Author: Anwar Khan. Email:
Computers, Materials & Continua 2025, 83(2), 3369-3388. https://doi.org/10.32604/cmc.2025.060474
Received 02 November 2024; Accepted 21 February 2025; Issue published 16 April 2025
Abstract
Traffic forecasting with high precision aids Intelligent Transport Systems (ITS) in formulating and optimizing traffic management strategies. The algorithms used for tuning the hyperparameters of the deep learning models often have accurate results at the expense of high computational complexity. To address this problem, this paper uses the Tree-structured Parzen Estimator (TPE) to tune the hyperparameters of the Long Short-term Memory (LSTM) deep learning framework. The Tree-structured Parzen Estimator (TPE) uses a probabilistic approach with an adaptive searching mechanism by classifying the objective function values into good and bad samples. This ensures fast convergence in tuning the hyperparameter values in the deep learning model for performing prediction while still maintaining a certain degree of accuracy. It also overcomes the problem of converging to local optima and avoids time-consuming random search and, therefore, avoids high computational complexity in prediction accuracy. The proposed scheme first performs data smoothing and normalization on the input data, which is then fed to the input of the TPE for tuning the hyperparameters. The traffic data is then input to the LSTM model with tuned parameters to perform the traffic prediction. The three optimizers: Adaptive Moment Estimation (Adam), Root Mean Square Propagation (RMSProp), and Stochastic Gradient Descend with Momentum (SGDM) are also evaluated for accuracy prediction and the best optimizer is then chosen for final traffic prediction in TPE-LSTM model. Simulation results verify the effectiveness of the proposed model in terms of accuracy of prediction over the benchmark schemes.Keywords
Cite This Article
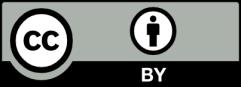
This work is licensed under a Creative Commons Attribution 4.0 International License , which permits unrestricted use, distribution, and reproduction in any medium, provided the original work is properly cited.