Open Access
ARTICLE
A Chinese Named Entity Recognition Method for News Domain Based on Transfer Learning and Word Embeddings
1 Naval University of Engineering, Wuhan, 430033, China
* Corresponding Author: Liangzhong Cui. Email:
Computers, Materials & Continua 2025, 83(2), 3247-3275. https://doi.org/10.32604/cmc.2025.060422
Received 31 October 2024; Accepted 04 March 2025; Issue published 16 April 2025
Abstract
Named Entity Recognition (NER) is vital in natural language processing for the analysis of news texts, as it accurately identifies entities such as locations, persons, and organizations, which is crucial for applications like news summarization and event tracking. However, NER in the news domain faces challenges due to insufficient annotated data, complex entity structures, and strong context dependencies. To address these issues, we propose a new Chinese-named entity recognition method that integrates transfer learning with word embeddings. Our approach leverages the ERNIE pre-trained model for transfer learning and obtaining general language representations and incorporates the Soft-lexicon word embedding technique to handle varied entity structures. This dual-strategy enhances the model’s understanding of context and boosts its ability to process complex texts. Experimental results show that our method achieves an F1 score of 94.72% on a news dataset, surpassing baseline methods by 3%–4%, thereby confirming its effectiveness for Chinese-named entity recognition in the news domain.Keywords
Cite This Article
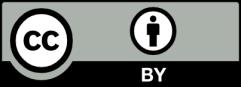
This work is licensed under a Creative Commons Attribution 4.0 International License , which permits unrestricted use, distribution, and reproduction in any medium, provided the original work is properly cited.